Here’s what works when your company’s data literacy increases
A few months ago, my friend Tim took a lead data science job at a media company that is transforming into a data driven company. He’s one of the best data scientists I know, but soon after starting, he emailed me to say he was struggling.
“We built a recommendation algorithm,” Tim said. “It works well, but no one in the company is using it.”
As I’ve written before, I love helping teams craft the high-level strategic story that gets their work out in the hands of business to have some serious impact. So Tim and I met for coffee at one of the Starbucks bars in Amsterdam to review his approach.
Sipping a large Caramel Macchiato I asked Tim: “At what point does management tune out?”
“Usually when explaining the problem they have,” he said.
“You,” I said, “talk like a technologist solving a problem. Management is concerned with business outcomes, not problems. Help them to be successful and you’ve got yourself a winner. And, start with the team…”
Five Elements of Successful Data Science Companies
The past years I have been part in many data science teams ranging from startups to multi billion companies. During this time I have observed the evolution of companies becoming more data driven; a journey to improve data literacy in the company, educating data scientists, data engineers, and analysts. Some of these companies succeeded. This is why.
#1 Immerse Data Scientists With the Business
Most companies start with a centralised model where everybody reports to a single department; often finance. This works when the team is relatively small, but becomes quickly unsustainable when the company grows rapidly. Analysts lose sight of what is critical to the business and it becomes increasingly difficult to “sell” your solutions. It then becomes obvious that the analyst has to be closer to the business in order to develop their functional expertise.
At many companies I consulted for, this is the time where they move towards a hybrid model where they have a functional analytics teams like marketing analytics, content insights or sales analytics, that are really focussing on driving functional impact. On top of that they still have a centralised team that is focussing on building internal data products and predictive algorithms.
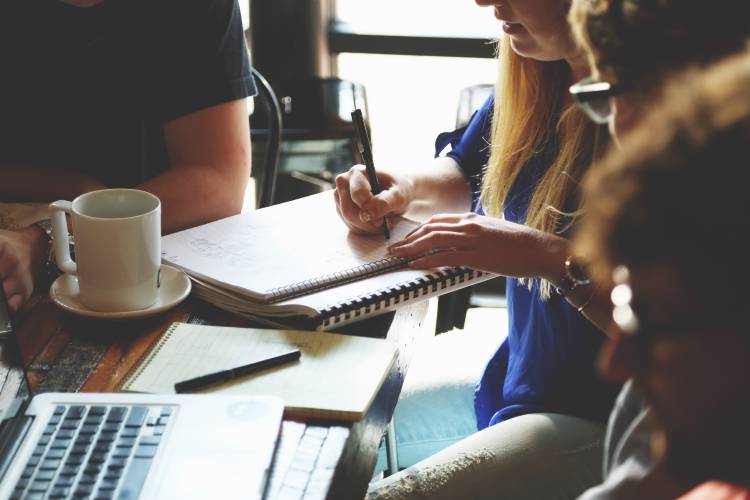
#2 Focus on Supporting Long Term Strategic Initiatives
Meanwhile you do need a team that is more focussed on some sort of the long term culture supporting strategic initiatives. It’s great to have a centralised team that is focussed on developing these long term products or developing different tools that can support analysts and data scientists. These teams are not involved in the day to day craziness of the business and would have the luxury to work an iterate on the processes, products and tools that can really make the live of the data scientist or data analyst easier and increase the companies data literacy.
#3 Support From the Senior Management Up To the CEO
That’s probably the most critical. We are not talking about moral support but the willingness to really carve out a significant portion of the budget to invest in the technology and the people. I have seen many companies fail because of being reluctant. What will happen is that that the talented people will ran out the door because they didn’t feel like the company is reallybeing data driven. They don’t feel its the place they can grow. And that’s when the whole thing starts to fall apart.
#4 Don’t Just Copy Other Companies
Lastly, I would say don’t just copy other companies. It’s very easy to think like: Oh look like how Facebook is organised, look like how they have setup their organisation; let’s just do the same thing. It is important to learn about the pros en cons of different models and ultimately you need to pick the model you believe works best for you, your organisation, and your budget.
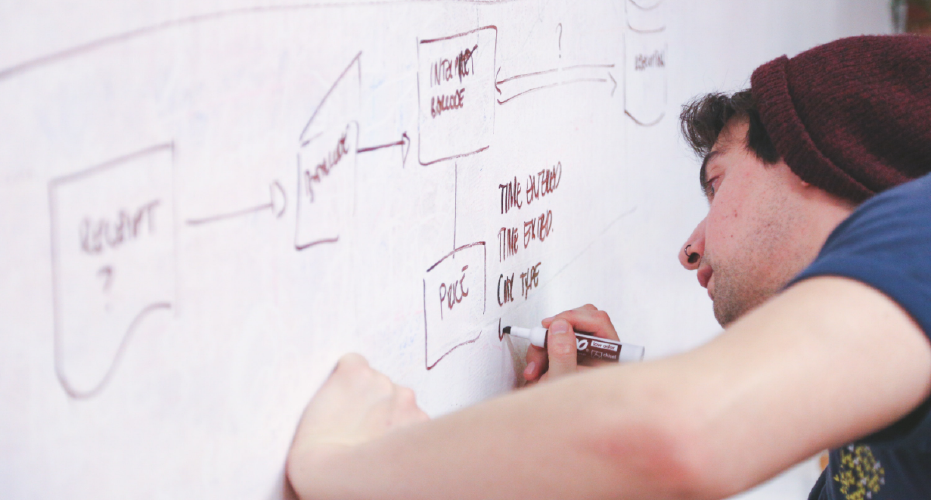
#5 Keep Iterating, Keep Improving
You also need to be constantly evaluating and adjusting if necessary. Like the previous example, the centralised model works fine for a while. Until the company starts to grow too fast. At that time changes need to be made. At Spotify they say “Change is the new constant” and that is probably even more true for data. The technologies in data science, machine learning, and AI are evolving rapidly and so we also need to continue to adapt and optimise our organisations so we can really drive the biggest impact from the data we have.
Do you think this was interesting, but you’re also convinced you can do better? That’s great, because then we want to talk to you! We’re hiring :) Have a look at our website @ https://primed.io/careers/ or send us an email on info@primed.io